We’re in a period of dizzying progress in “AI” on all fronts: no day goes by without startling advances in computer vision, robotic control and natural language processing. Above all, Large Language Models (LLMs) have turned our assumptions on their head about what is possible for a computer to achieve. Humanoid robots give us the impression that artificial human companions will soon be flooding our factories and homes to help us in work and in play. It’s impossible to keep up with the deluge of new papers. Artificial General Intelligence (AGI) is hurtling towards us. The future is almost here.
It’s hard not to be optimistic. It’s also hard not to be cynical, particularly if you’ve been ground down by the reality of as hard a discipline as robotics. Are LLMs really intelligent? Are they on a path to sentience, and what does that even mean? Are humanoid robots practical? If “artificial intelligence” feels nebulous at times, “artificial general intelligence” is even more so. I lurch back and forth between optimism, as I see stunning advances in what we can do and cynicism, as I see overblown claims for where we are.
It seems to me that what we have right now is the ability to build new, amazing, unprecedented tools to accomplish particular tasks: AI vision (“segment this image on a pixel by pixel basis”), reinforcement learning (“learn a policy to pick up widgets using trial and error”) and LLMs (“use your massive general knowledge to generate new text”). We do not have a coherent vision for putting these marvellous tools together into something with the flexibility and tenacity of a lifeform. We have many single-purpose parts. We do not have an architecture. This lack of a coherent framework for complete real-world agents is the first core problem in AGI today.
The second core problem is that, because of its success and ubiquity, we tend to see digital computing as the only substrate for building these new creatures. We miss the importance of hardware, of embodiment; the shape of a fish fin outweighs any amount of clever code when swimming. We miss the power of low-level analogue electronic circuitry and it’s equivalent in animal reflexes and spinal cords. We miss the role of the environment, dismissing it as a nuisance or simply as one more annoying problem to be solved, until it resurfaces in bizarre research where artificial octopus tentacles in a fish tank appear to solve mathematical problems. We’re overspecialised, and each discipline (fluid mechanics, physiology, ethology, mechanical engineering…) only rarely peers over the wall into its neighbours’ garden. Where are the unifying principles?
Third, where is the intentionality in our new tools? Each application is built to achieve a single goal: to predict how proteins are folded, to generate text, to analyse an image. Reinforcement learning, the most explicitly goal-driven framework we have is still generally tied to a single goal. It “wants” to learn to pick up a vegetable with a robot hand, it’s not trying to ensure the survival of its agent.
Into all this promise, and all this confusion, strolls a quiet, pipe-smoking English professor who died back in 1970, who spent decades thinking through these problems before the widespread availability of digital computing, who built a machine that nobody understood and who wrote the single best book I’ve ever read on the subject of artificial intelligence. Ladies and gentlemen, please meet W. Ross Ashby.
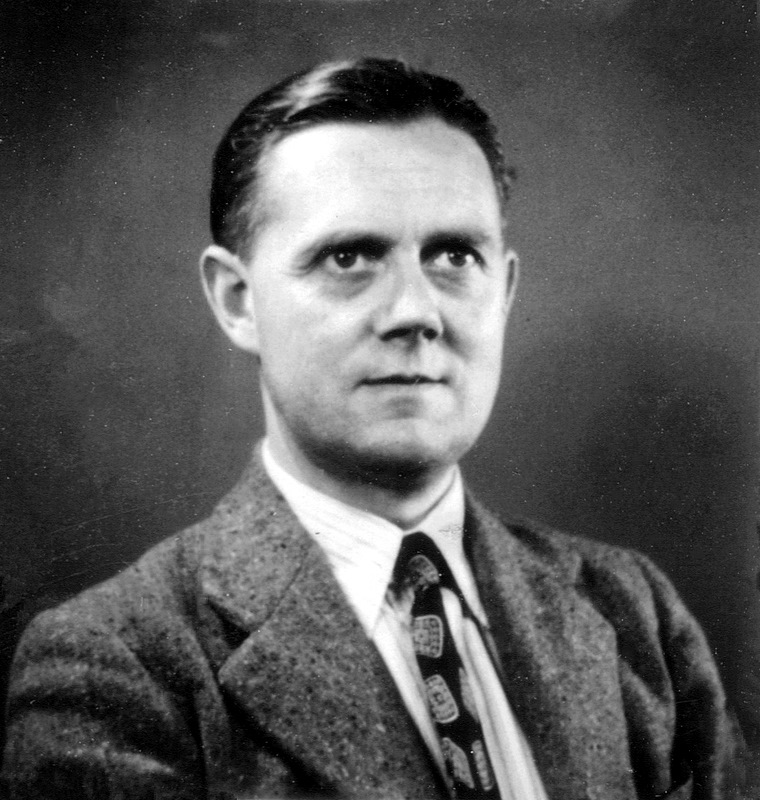
Cometh the Hour, Cometh the Man
Ashby was a clinical psychiatrist, a mathematician and a cyberneticist. He repaired watches. He painted. He pottered in his shed, building strange devices. Ashby was obsessed with questions like, how could the brain work? What are the simplest mechanisms by which a lifeform can adapt to its environment? What does a high-level animal such as the human have in common with a slime mould? Working when he did, he didn’t have the option of easy answers such as “a computer program” or “a neural network”. He had to really think.
From the 1940s to the 1960s, cybernetics was the study of complex, feedback-driven systems and considered to be a discipline that could be applied to engineering, biology, management and economics. Ashby was member of a dining club of engineers, mathematicians, biologists, physiologists and psychologists. They were actively looking for general solutions applicable to many types of problem, outside of their own siloes.
Ashby framed his chosen problem as one of adaptive behaviour; how can a system (such as, but not limited to a lifeform) adapt to a changing environment? The first iteration of his solution was published in 1941, reached its apotheosis in his astonishing book, “Design for a Brain” in 1952/1967 (get the 2nd edition) and was still in development at his death in 1970. His framework encompasses subjects that would now be taught as many separate university courses: dynamical systems, causal networks, embodied intelligence, feedback control and so on.
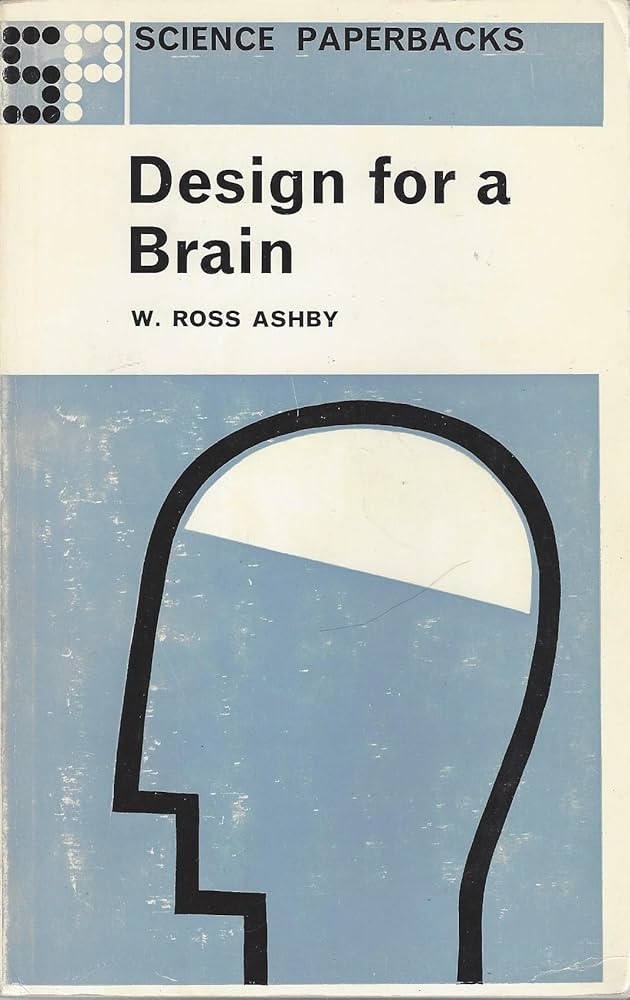
His books are beautifully written and an absolute model of clarity. In a masterstroke, he relegates all the mathematics in “Design For a Brain” to an appendix, widening the potential readership from a sophisticated researcher to a patient high school student. Doing this also forced him to explain his ideas clearly without hiding behind equations. For anyone who has had to trudge through modern research papers that obscure very marginal contributions behind a wall of obfuscatory maths, reading Ashby is a joy.
So what did he say?
Ashby’s Framework for Adaptive Behaviour
Ashby thought in terms of machines; a machine being a set of variables that evolves in some predictable way from a given starting point. For a programmer, this could be a state machine; for an engineer, a dynamical system like a pendulum; for an economist, a countries’ economy. In each case, the machine is an abstraction and simplification of an underlying reality and represents a choice: depending on which variables you choose to measure, you may abstract an infinite number of different systems, only some of which form predictable, deterministic machines. The core principle of cybernetics is that you can work with these abstractions, whatever particular discipline the problem comes from.
Here is how Ashby saw an adaptive system or agent:
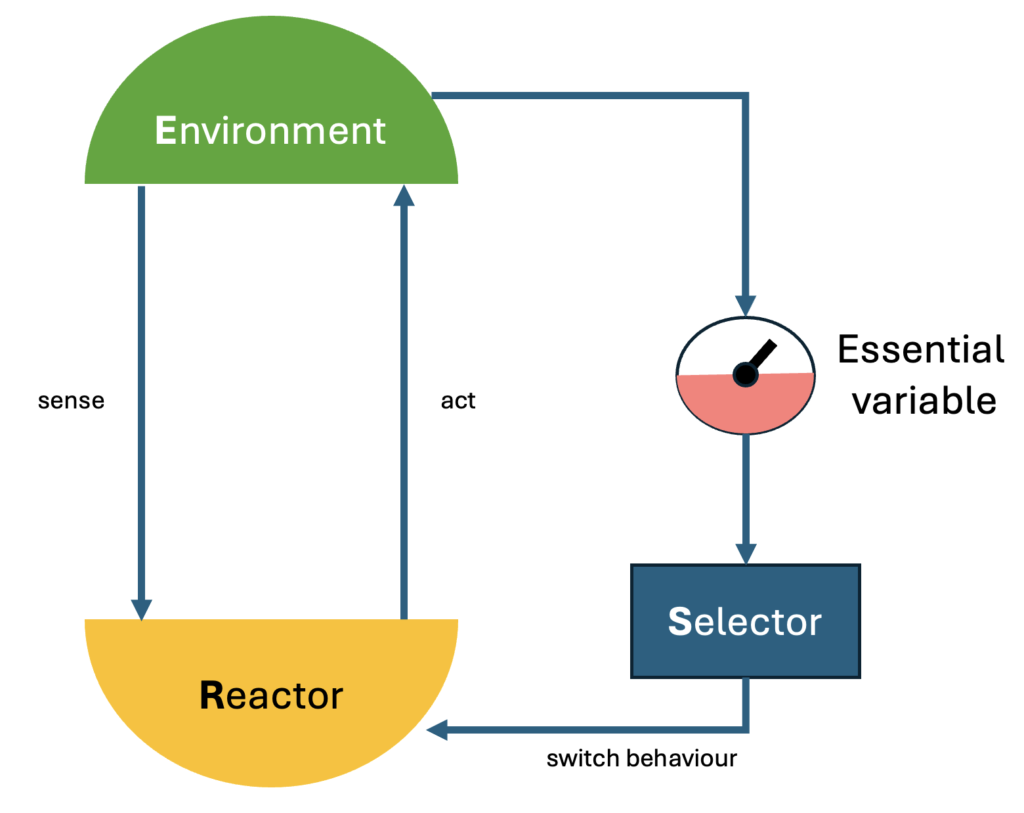
To over-simplify, the Reactor is a machine that abstracts the bulk of the agent. It interacts with its Environment as one might expect, by sensing and acting. It shows an identifiable behaviour. The Reactor is constructed so as to behave in a way that handles minor fluctuations and disturbances in the environment. The environment acts on Essential Variables that are critical to the agent’s well-being, think “hunger” or “body temperature”. While these essential variables are kept by the Reactor within critical ranges (well-fed, not too hot, not too cold), then all is well. When however, an essential variable leaves its critical range (“I’m too hot!”), then another machine, the Selector, responds to this and randomly selects a new behaviour from the Reactor. If the new behaviour returns the essential variables to where they should be then this selection persists. If not, after a short while the Selector makes a new random selection and the agent tries out another behaviour. This, says Ashby, is the root of all adaptive behaviour.
In itself, this doesn’t sound like much to write home about. How many lines of code is that? Bear with me.
There are two feedback loops at play here: an “inner” one between Reactor and Environment that operates continuously, and an “outer” one that switches between behaviours when necessary. Ashby provides crisp, precise definitions of “machine”, “behaviour” and all the other terms I’m using very loosely in this overview. Most interestingly for us, the Reactor, Selector and Environment are abstractions, mere sets of variables and can be implemented in any dynamical system: software, hardware, wetware or pure maths. The underlying nature of the “module” is less relevant than its role; we can unite digital and analogue tools with hardware in a single framework. It provides a method for decomposing complex, living creatures into systems that can be measured and studied a quantified manner, and provides a blueprint for building new ones.
Show Me the Beef
Ashby gave many illustrative examples, including one of a kitten that “learns” not to touch a glowing ember from a fire. I’d like to give my own modern example, more applicable to robotics.
Until recently, humanoid robots have been notoriously bad at walking. Simultaneously controlling and coordinating all the joint actuators in a pair of legs, such that the robot does not fall over, is decidedly non-trivial. One approach that doesn’t come naturally to coders, is to first do as much as possible in hardware. The extreme example of this is the passive walker: a mechanical pair of legs built with springs and weights. No motors, no electricity, no computing. If you place a passive walker at the top of an inclined plane, it will walk down the ramp, driven purely by gravity, and can even step over and compensate for small obstacles such as stones. Here is a physical example of Ashby’s Reactor. It acts on the Environment and through physical feedback can quickly adapt to minor disturbances. This is Ashby’s “inner loop” between Reactor and Environment.
The passive walker is a tool for walking; how can we make it a complete adaptive agent? Let us suppose that for good reasons, the passive walker is afraid of lions. If an Essential Variable is “proximity to the nearest lion” and should be kept above a minimum value, what would a Selector look like?
To keep the example as non-digital as possible, let’s assume that the agent has a rudimentary chemical nose that can smell lions, and this provides it with an estimation of the proximity variable. Let’s also assume that this nose releases a hormone that, perhaps through the medium of some sort of blood-like liquid, can affect the stiffness of the springs in the passive walker. By altering the walker’s spring stiffnesses you can switch it into a new gait. Depending on the values of hormone selected, the walker can begin to run. As it runs, the walker escapes the lion, and the “proximity” variable returns to a safe value. This is the second, “outer” feedback loop.
Gaits are discrete, identifiable behaviours (running, walking, skipping etc.) although the underlying dynamical mechanism is continuous. The agent doesn’t have to know which gait to jump to (although evolution could eventually hard-wire this), it simply has to switch randomly, try for a number of seconds, and then change again if its variable isn’t back within its comfort zone. Even with random selection, the agent will quickly find and settle on the running gait as the number of discrete gaits is small. I suspect that such an agent could be built with springs and chemicals, without a single piece of digital electronics, let alone a GPU. It could also be a simulation in Python, but the principle, the system, the abstract “machine” would be the same.
Implications
It’s not magic. At every step of his argument, Ashby is careful to show that such “ultrastable” systems require no extra suppositions, no unexplained mechanisms, no homunculi, no ghosts in the machine.
He addresses the scaling and complexity problem: what happens if there are multiple Essential Variables (“proximity to lion”, “tiredness”, “thirst” etc.)? Can such systems settle on adaptive behaviours that control for everything simultaneously in a biologically realistic timeframe? In one of his most evocative results, he shows that they can, providing that the environment shows certain regularities (and real environments do) and that the Reactor/Selector (in the loosest sense) is compartmentalised. The brain, Ashby says, must be highly interconnected to achieve useful behaviours, but not too interconnected, or it will take too long to arrive at stability. Each Essential Variable and Selector become semi-independent, communicating with each other largely through the medium of the Environment:
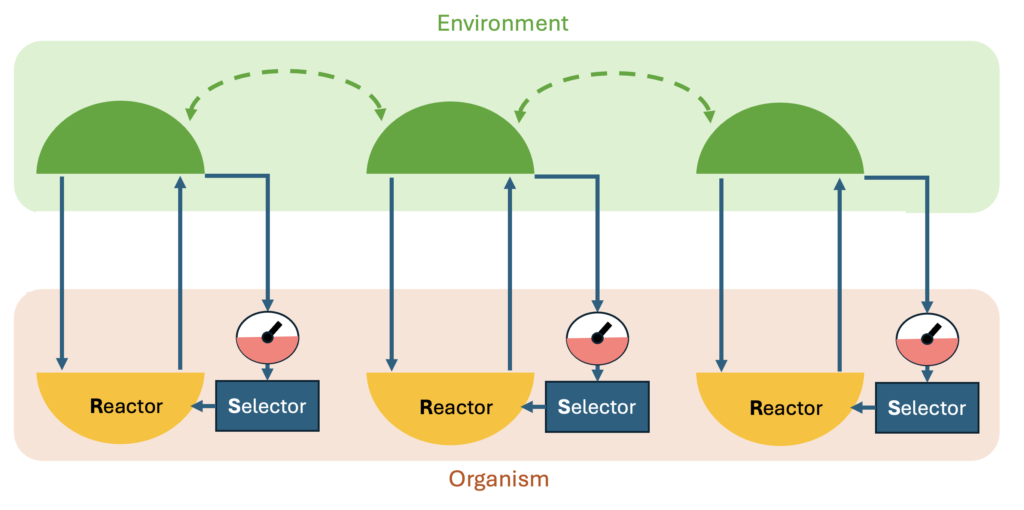
We see this in nature: the brain is compartmentalised to a high degree. We see it in robotics: hexapods converge on a walking gait as the independent legs interact with each other through the environment. We see it in the computational efficiency and power of sparse neural networks.
Going deeper, Ashby shows how his framework can be hierarchical; the above diagram can represent a whole lifeform, or merely one part of the brain, where the rest of the creature acts as the “Environment” for the part being examined. He shows how behaviours can be “remembered” (though no memory as such is involved), rather than randomly re-sampled each time.
Ashby’s thought-experiments are meticulous and inspiring. But he was also a builder.
The Homeostat
To demonstrate his theories, he constructed a machine called the Homeostat. It was a gorgeously analogue device, with four connected “units” made from water-filled potentiometers, each of which could affect all the others. Each unit represented an Essential Variable plus Selector and could change the machine’s behaviour when it left its critical range.
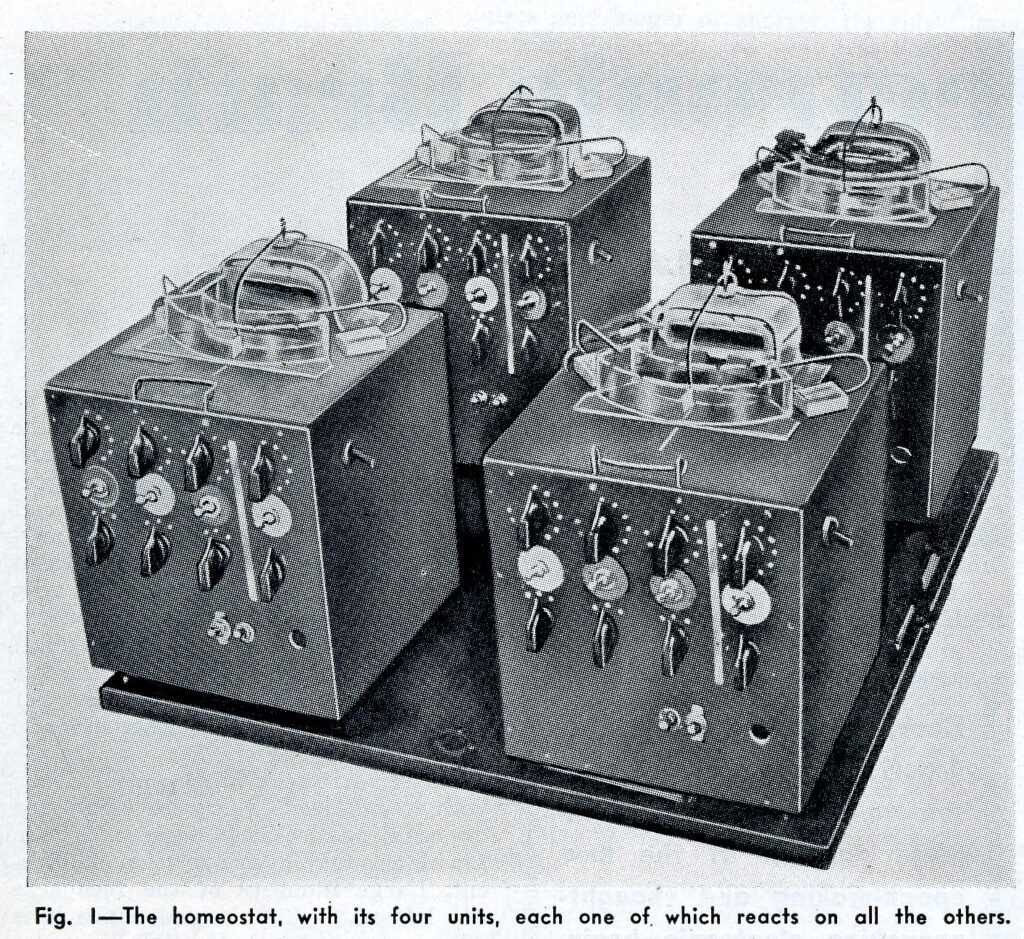
The upshot was, if you fiddled with the controls the machine would go a little nuts and waggle its potentiometers before settling on a new, stable configuration. Ashby used this to verify many of the logical conclusions he’d deduced from his framework. It was at once brilliant, eccentric and in the long term probably counter-productive to the acceptance of his ideas.
“It may be a beautiful replica of something, but heaven only knows what,” said fellow cyberneticist, Julian Bigelow. Others just scratched their heads. What was this thing for? It didn’t look much like an intelligent machine.
Another persistent criticism from his peers was that Ashby’s framework suggested a world of passive creatures, reacting only to occasional external disturbances before settling back into stasis. William Grey Walter called the homeostat a “sleeping machine”, like a dog sleeping by the fire, that reacts only to the occasional prod before sinking back into a stupor. To me, this objection disappears as soon as you consider a realistic list of Essential Variables in any higher-level organism. What if the dog gets hungry and there’s nobody there to feed it? It is unlikely to behave so passively then.
But I believe the principal reason that Ashby’s work is largely forgotten today is that it was published just in time to be steamrollered by the 1970s digital computing revolution. Suddenly, there were exciting new avenues for “AI” such as symbol processing and deep grammars. Ashby and his “useless machine” were yesterday’s news. Cybernetics itself vanished off the map as its originators retired or died, and the subject became dispersed in countless siloed university courses: dynamical systems, causality, feedback control, complex networks… The search for general solutions and general frameworks was lost in the rush to build tools with the new technology.
¡Viva Cybernetics!
As I’ve said elsewhere, I have a dangerous tendency to romanticise forgotten, out-of-fashion research. It seems far more exciting to unearth buried treasure and polish it than to stick to the hard slog of constructing something new, piece by piece. You’ve got LLMs, you say? Let me tell you about the Subsumption Architecture! Caveat emptor. You should view this article through that filter.
Nevertheless, I believe that Ashby’s work, and cybernetics more generally, address key deficiencies in the way we pursue “AI”. It is a high-level abstract framework that unifies very different ways of implementing solutions to real-world tasks. It shows intentionality. It doesn’t depend on GPUs or back-propagation, but can easily encompass both. It dovetails perfectly with research into learning and into evolution. The closest parallel I can find in today’s research is Friston’s Free Energy Principle, which posits a mathematical framework for life. Yet for me at least, Friston’s publications are hard to read and digest, and overly-drenched in mathematics. They are tantalising, but it’s unclear to me if his principle is one of the most important advances since Darwin, or just a mathematical anecdote. I read Friston, and I don’t know what to build. The major scientific work that has survived usually originates in extremely well-written books; try reading Galileo, Darwin or even Freud. Every page will elicit an “I get it!” Ashby too is crystal clear, simple to follow and makes me ache to get back to the keyboard and soldering iron.
I don’t believe anyone interested in AGI can afford to ignore Ashby.
You should order yourself a copy of “Design for a Brain” right away.